Give Your AI Apps the Context They Lack
Personalized AI with Context
GraphRAG pipelines feed LLMs by combining search techniques and graph algorithms. You get accurate, context-rich answers that go beyond the generic LLM outputs everyone defaults to since their models were trained on the same public data.
Vectors and Graphs joined forces!
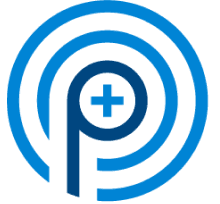
Why GraphRAG with Memgraph?
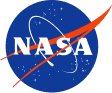
Memgraph Tools to build GraphRAG
Memgraph as Your Context Engine
Use Memgraph as the backbone for your GraphRAG apps. It's built for scale, performance, and can handle complex queries across large knowledge graphs—whether you're working with millions of nodes or performing real-time calculations.
Vector Search
Vector search is a new feature that combines the strengths of similarity search with relevance-based graph search, enabling efficient and precise information retrieval within graph-based datasets.
Integrated Algorithms
Memgraph's powerful algorithms—Community Detection (Louvain, Leiden), PageRank, and Graph Traversals—ensure your AI isn't just making things up. It responds based on real, structured relationships embedded within your data.
GraphChat
Query your graph with plain English. GraphChat is your direct line to your graph database inside Memgraph Lab. Forget about writing complex queries—just ask. GraphChat translates your natural language question into a Cypher query, runs it on Memgraph, and provides you with the best possible answer in human language.
This two-phase generative AI app gives you answers grounded in the context of your knowledge graph.
LangChain and LlamaIndex Integrations
Memgraph integrates with Langchain and LlamaIndex, allowing for multi-hop retrieval to answer complex questions by connecting data from different sources. You can easily integrate Memgraph with your existing LLM workflows to power advanced knowledge extraction.
Upcoming Features
Reasoning Graph: AI That Picks the Right Tools for the Job
Memgraph isn't just storing data—it's thinking with it. Reasoning Graph removes the guesswork by automating search and retrieval.
It analyzes query intent and dataset structure, dynamically choosing the best method—vector search, graph traversal, or multi-hop reasoning—so AI gets the right answers without developer intervention.
Looking for a specific feature to build your GenAI-app?
Let us know what you're missing.