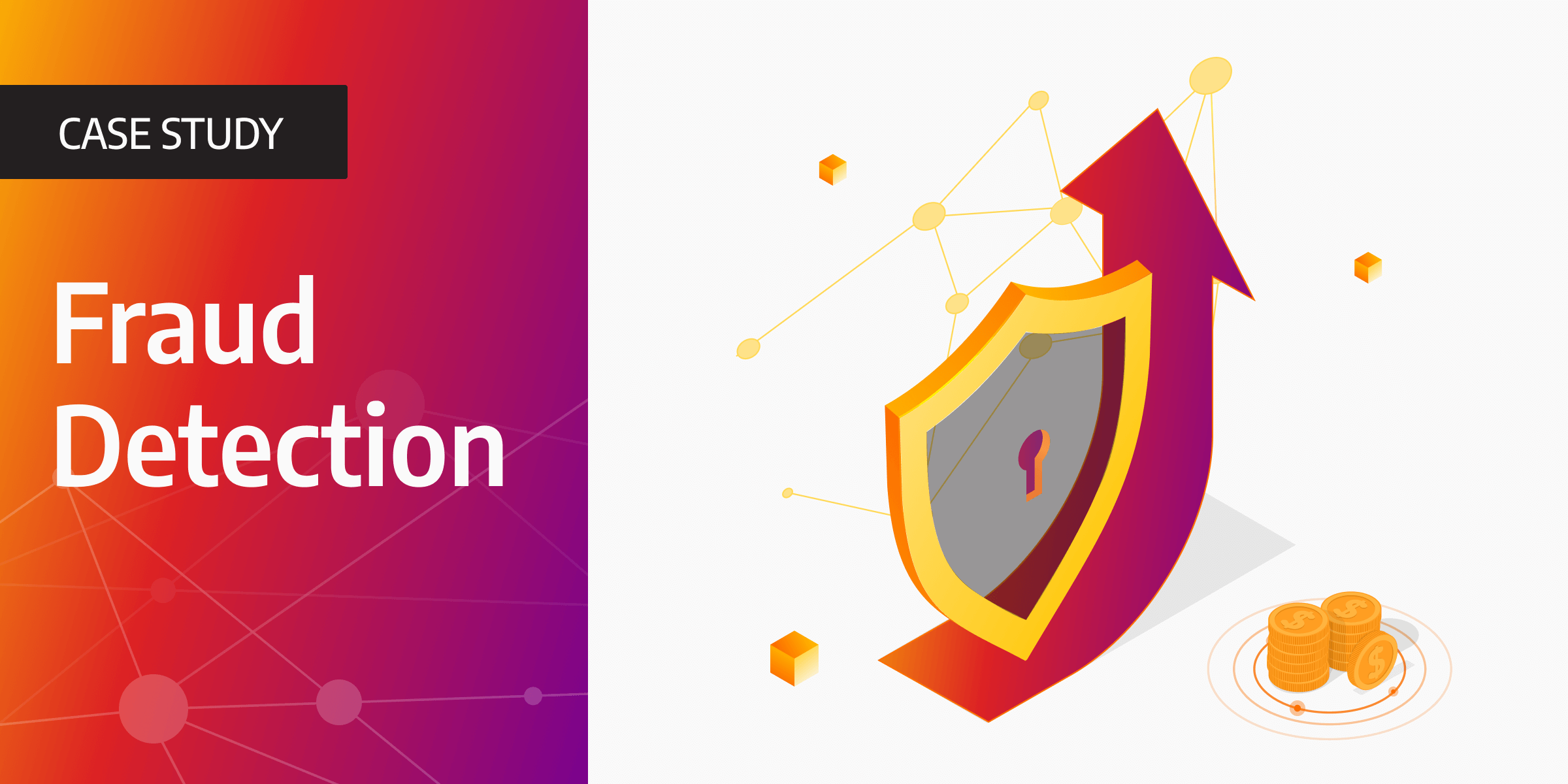
From Losses to Savings: How Memgraph Helped Company X Save 7 Figures through Fraud Detection
Graph technology in fraud detection has proved to be massively effective due to the interconnected nature of fraudulent activities. Fraud-related data is rich in relationships, yet tackling fraud detection requires analysis beyond individual entities and consideration of the bigger picture. With this in mind, Memgraph and Company X have come together to address the issue of fraud in one of the leading insurance providers headquartered in Europe, with offices across the US.
In this case study, you will learn how the collaboration between Memgraph and Company X yielded impressive results, demonstrating that incorporating graph analytics into the existing ML models greatly enhanced fraud detection effectiveness. By merging the capabilities of graph technology with the existing models, Company X achieved the following results:
- Significant increase in fraud detection across various types of claims.
- 135% increase in detection efficiency.
- Substantial savings in the seven-figure range for over a million claims processed.
Company X and Memgraph joint efforts
Company X, a prominent global insurance provider, is renowned for its comprehensive range of insurance products, including auto, home, life, health, and business coverage. They prioritize customer satisfaction and employ personalized approaches, incorporating innovative tools and digital platforms to streamline operations and improve the insurance experience.
To tackle the significant challenge of fraud detection, Company X aimed to utilize graph analytics to unveil previously undetected fraud by connecting diverse data silos and leveraging data relationships. Given the higher prevalence of fraud in the United States, Memgraph collaborated directly with the Claims Department at one of Company X's US offices.
From rules engines to graph technology
Insurance fraud presents a significant challenge to the industry, involving deceptive practices aimed at gaining financial benefits through fraudulent means. The exact extent of insurance fraud in the United States is difficult to quantify due to its diverse nature and potential underreporting. However, it imposes substantial costs on both insurance companies and consumers.
Estimates from the Coalition Against Insurance Fraud (CAIF), a nonprofit organization dedicated to combating insurance fraud, indicate that fraudulent activities cost the insurance industry billions of dollars annually in the United States. These activities include fraudulent claims, premium evasion, and fraudulent applications, resulting in losses ranging between $80 billion and $120 billion per year, as reported by the CAIF.
Traditionally, the insurance industry relied on rules engines for fraud detection. However, as systems grew more complex and fraudsters became more sophisticated, rules engines faced limitations in identifying broader patterns or contexts. Graph technology emerged as a valuable solution, leveraging the interconnected nature of insurance data to enhance fraud detection capabilities.
Memgraph as the solution
Company X explored the graph technology landscape and selected Memgraph as their partner to achieve their fraud detection objectives. Memgraph’s in-memory graph analytics capabilities empower users to derive insights and tackle complex operations, making it an ideal tool for solving intricate problems in various domains, especially fraud detection scenarios with highly interconnected data networks.
Collaborating with Company X, Memgraph integrated a fraud detection model into their existing system, combining rules, machine learning, and graph technology. Memgraph's objective was to demonstrate how graph analytics could uncover previously undetected instances of insurance fraud by connecting data silos and leveraging data relationships. To that end, the following goals were established:
- Identify fraudulent claims missed by traditional methods
- Increase the quality of Special Investigation Unit referrals
- Decrease losses paid on potentially fraudulent claims
The existing fraud detection system of Company X operated on rule-based methods. Memgraph's graph technology was introduced to enhance the existing system by identifying fraudulent claims beyond those already detected through traditional approaches. Company X had a robust system for investigating flagged claims, but it struggled with a high rate of false positives—claims that initially seemed fraudulent but were proven legitimate upon investigation. Thus, Memgraph's objective was to enhance the accuracy of referrals made to the investigation system by reducing the occurrence of false positives. Achieving these objectives would ultimately lead to a decrease in losses paid out for potentially fraudulent claims.
Collaboration outcomes
_Head of Advanced Analytics at Company X, noted that using Memgraph could have saved them over seven figures in fraud prevention on similar datasets. With Memgraph's integration, Company X saw a 135% boost in detection efficiency across various claim types, resulting in significant seven-figure savings from over a million processed claims. Overall, the partnership with Memgraph proved highly effective in detecting and preventing both fraudulent and legitimate claims, helping Company X reduce financial losses and protect their assets.
For a full, detailed insight into how this Company X used Memgraph, download the customer story.